One of the most important
actions that can help maintain the quality of any good or
service is to collect relevant data consistently over time,
plot it, and examine the plots carefully. All statistical
process control charts plot data (or a statistic calculated
from data) versus time, with control limits designed to
alert the analyst to events beyond normal sampling
variability.
STATGRAPHICS Centurion
provides a very extensive collection of control charts.
These include:
1.
Basic charts for
variable data in which each point
represents the most recent data, including X-Bar and
R charts, X-Bar and S charts, X-Bar and
S-squared charts, Median and Range charts,
and Individuals charts based on X and MR(2).
2.
Basic charts for
attribute data, including P, NP, U, and C
charts.
3.
Time-weighted charts
in which the points plotted are calculated from both
current and historical data, including MA, EWMA, and
CuSum charts.
4.
Multivariate charts,
designed for situations where multiple correlated
measurements are collected.
5.
ARIMA control charts for autocorrelated
data in which the samples collected from one time period
to the next are not independent.
6.
Toolwear charts for monitoring data that
is expected to follow a trend line, not remain constant
at a fixed level.
7.
Acceptance control
charts for high Cpk processes, where the
control limits are placed at a fixed distance from the
specification limits rather than the centerline of the
chart.
8.
CuScore charts, which are designed to
detect specific types of patterns when they occur.
All control charts can be
used for Phase I studies, in which the data determine
the location of the control limits, and Phase II studies,
in which the data are compared against a pre-established
standard. A special procedure is also provided to help
design
a control chart with acceptable power.
Basic Variables Charts
The classical type of
control chart, originally developed back in the 1930's, is
constructed by collecting data periodically and plotting it
versus time. If more than one data value is collected at the
same time, statistics such as the mean, range, median, or
standard deviation are plotted. Control limits are added to
the plot to signal unusually large deviations from the
centerline, and run rules are employed to detect other
unusual patterns.
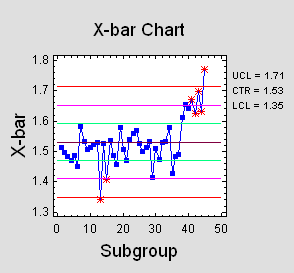 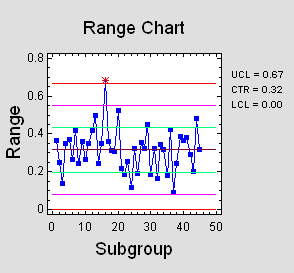
Basic Attributes Charts
For attribute data, such
as arise from PASS/FAIL testing, the charts used most often
plot either rates or proportions. When the sample sizes
vary, the control limits depend on the size of the samples.
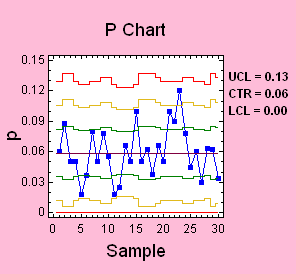
Time-Weighted Charts
When data is collected
one sample at a time and plotted on an individuals chart,
the control limits are usually quite wide, causing the chart
to have poor power in detecting out-of-control situations.
This can be remedied by plotting a weighted average or
cumulative sum of the data, not just the most recent
observation. The average run length of such charts is
usually much less than that of a simple X chart.
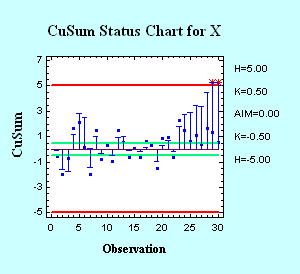
Multivariate Control Charts
When more than one
variable are collected, separate control charts are
frequently plotted for each variable. If the variables are
correlated, this can lead to missed out-of-control signals.
For such situations, STATGRAPHICS provides several types of
multivariate control charts: T-Squared charts, Generalized
Variance charts, and Multivariate EWMA
charts. In the case of two variables, the points may be
plotted on a control ellipse.
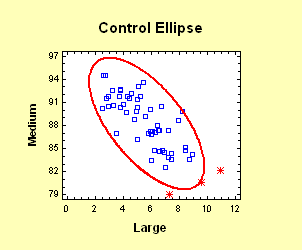
ARIMA Control Charts
With today's automated
data collection systems, samples are frequently collected at
closely spaced increments of time. Any sort of process
dynamics introduces correlation into successive
measurements, which causes havoc with standard control
charts that assume independence between successive samples.
In such cases, a control chart that captures the dynamics of
the process must be used to properly detect unusual events
when they occur.
The proper chart for such
situations is often an ARIMA control chart, which is
based upon a parametric time series model for process
dynamics. Such charts either plot the residual shocks to the
system at each time period, or they display varying control
limits based upon predicted values one period ahead in time.
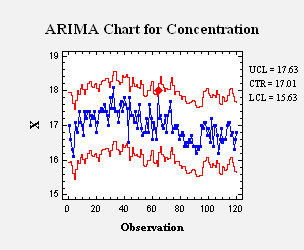
Toolwear Charts
Control charts can also
be used to monitor processes in which the mean measurement
is expected to change over time. This commonly occurs when
monitoring the wear on a tool, but also arises in other
situations. The control charts for such cases have a
centerline and control limits that follow the expected
trend.
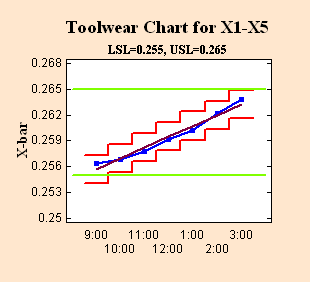
Acceptance Control Charts
For processes with a high
Cpk, requiring the measurements to remain within 3 sigma of
the centerline may be unnecessarily restrictive. In such
cases, the process may be allowed to drift, as long as it
does not come too close to the specification limits. A
useful type of control chart for this case is the Acceptance
Control Chart, which positions the control
limits based on the specification limits rather than the
process mean.
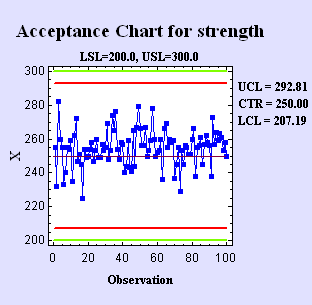
Cuscore Charts
When monitoring a
real-world process, the types of out-of-control situations
that are likely to occur may be known ahead of time. For
example, a pump that begins to fail may introduce an
oscillation into the measurements at a specific frequency.
In such cases, specialized CuScore Charts may be
constructed to watch for that specific type of failure.
STATGRAPHICS will
construct CuScore charts to detect: spikes, ramps,
bumps of known duration, step changes, exponential
increases, sine waves with known frequency and phase, or any
custom type of pattern that the user wishes to specify.
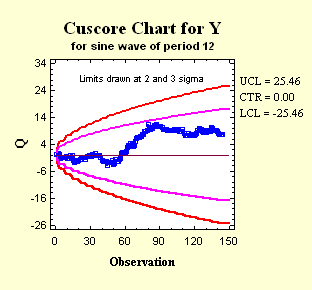
Control Chart Design
For a control chart to be
effective, it must be able to distinguish between situations
in which the process is operating as expected and those in
which it has deviated seriously from its target values.
STATGRAPHICS Centurion provides a procedure for designing
control charts that will detect deviations of a specified
magnitude within an acceptable time. In a typical
application, the user specifies a target mean and the
desired average run length before a deviation of that
magnitude is detected:
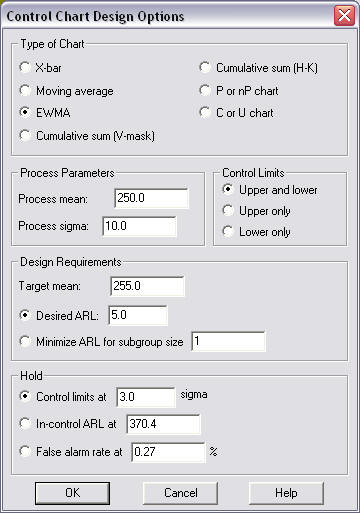
The procedure then
determines the number of samples and smoothing parameter
that will achieve the desired performance.
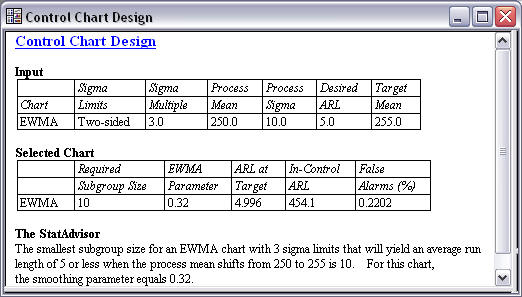
|