STATGRAPHICS contains
extensive capabilities for the creation and analysis of
statistically designed experiments. The designs that can be
created are divided into several types:
- Screening - designs
intended to determine the most important factors affecting
a response. Most of the designs involve only 2 levels of
each factor. The factors may be quantitative or
categorical. Included are 2-level factorial
designs, mixed level factorial designs, fractional
factorials, irregular fractions, and
Plackett-Burman designs. For designs of less than
full resolution, the confounding pattern is displayed.
Blocking and randomization are options.
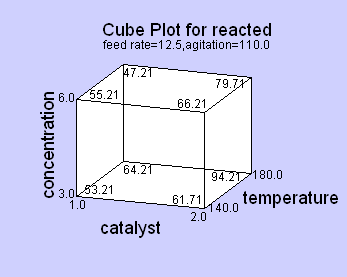
-
Response
Surface - designs intended to determine the
optimal settings of the experimental factors. The
designs involve at least 3 levels of the experimental
factors. Included are central composites, Box-Behnken
designs, 3-level factorials, and
Draper-Lin designs.
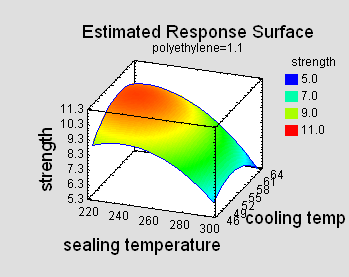
- Mixture
- designs involving components of a mixture, where the
levels of the components are constrained to sum to 100%
(or some other fixed value). Upper and lower constraints
may be specified for each component. Included are
simplex-lattice, simplex-centroid, and
extreme vertices designs.
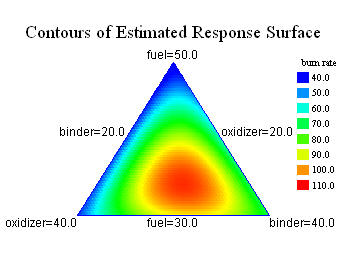
-
Multilevel Factorial - designs involving
different numbers of levels for each experimental
factor. These designs produce a natural candidate set
for the D-Optimal design creation procedure,
which will select an optimal subset of the runs.
-
Inner/Outer Arrays - designs consisting of both
controllable and uncontrollable (noise)
factors, intended to find combinations of the
controllable factors at which the responses are
relatively insensitive to the uncontrollable factors.
Following the methods of Genichi Taguchi, both an
inner and outer array are constructed. The factors may
be quantitative or categorical. As part of the analysis,
signal-to-noise ratios may be constructed.
-
Single
Factor Categorical
- designs intended to compare
levels of a single non-quantitative factor. Includes
completely randomized designs, randomized block
designs, balanced incomplete block (BIB) designs,
Latin Squares, Graeco-Latin Squares, and
hyper-Graeco-Latin Squares.
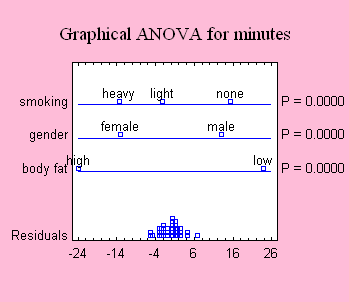
-
Multi-Factor Categorical
- designs intended to study multiple non-quantitative
factors, with several levels of each. Analyzed using a
multifactor analysis of variance.
- Variance
Component (hierarchical) - designs intended to
study the effect of two or more nested factors on the
variability of a response. Estimates of the contribution
of each factor to the overall variability are obtained.
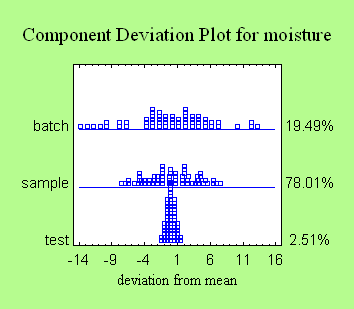
Major Steps in Constructing and Analyzing
an Experimental Design
Step 1: Create Design -
The experiment is created by completing a sequence of dialog
boxes. On these dialog boxes, the user specifies the
experimental factors and responses, the experimental region,
the order of randomization, and any blocking variables.
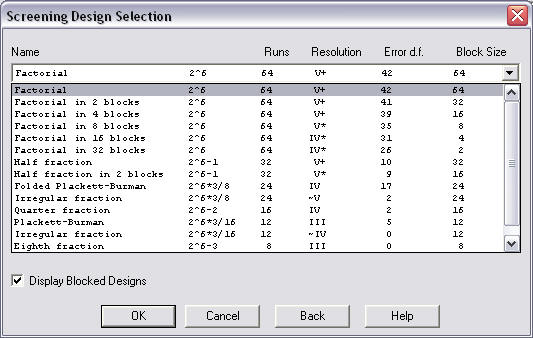
Step 2: Perform Experiment -
The selected experimental runs are then performed and the
responses entered into the experiment datasheet.
Step 3: Analyze Experiment -
The response variables are analyzed and a suitable
statistical model is developed. Usually, the principle of
parsimony is applied to remove insignificant effects
from the model.
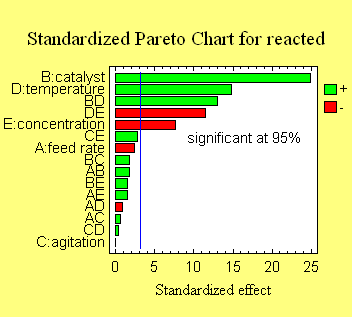
Step 4: Augment Experiment - If
necessary, additional runs are added to the initial design.
STATGRAPHICS provides facilities for adding runs to resolve
confounding, following the path of steepest ascent,
and adding star points to convert a screening design
to a response surface design.
Step 5: Optimize Response(s) -
Settings of the experimental factors are found that achieve
the desired responses. If more than one response is
present, desirability functions may be defined to combine
the different goals.
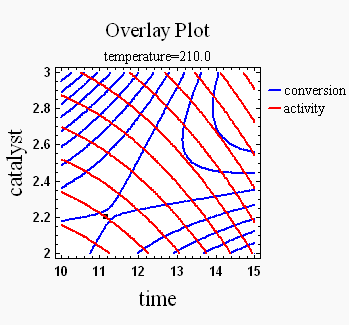 |