Determining the
reliability of manufactured items often requires performing
a life test and analyzing observed times to failure. Such
data is frequently censored, in that some items being tested may
not have failed when the test is ended. In addition, it may
be necessary to accelerate failure times by changing the
value of an influential variable such as temperature. For
all of these reasons, special tools are needed to deal with
this type of data.
STATGRAPHICS Centurion
provides several special procedures for dealing with
failures times:
1.
Life tables -
nonparametric methods for estimating the survivor
function (the probability that an item will still be
working at time t, as a function of t).
2.
Distribution fitting with
censored data - estimation of probability
distributions when the data contains censored
observations.
3.
Weibull analysis - special
techniques for fitting the very commonly used Weibull
distribution.
4.
Arrhenius plot - widely
used plot for an accelerated life test when the
accelerating variable is temperature.
5.
Life data regression -
estimation of a regression equation for life data with a
specified parametric form for the error distribution.
6.
Cox proportional hazards - estimation of a
regression equation for life data without specifying a
specific error distribution.
Life Tables
In analyzing life data,
interest commonly centers on estimating the probability that
a unit will still be operating at any given time. A common
way of estimating this survival function, without making any
assumption about functional form or error distribution, is
to tabulate the data and calculate the survivor function
directly from the observed failures. When censoring is
present, the estimates are calculated using the Kaplan-Meier
approach.
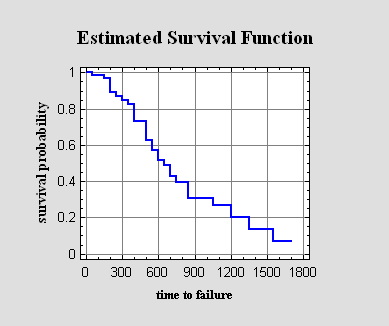
Distribution Fitting with
Censored Data
If sufficient data is
available, it may be possible to fit a specific distribution
to the failure times. Maximum likelihood methods can be
easily adapted to the presence of censored data.
STATGRAPHICS Centurion will automatically fit up to 45
probability distributions for any sample of data and rank
them according to goodness-of-fit.
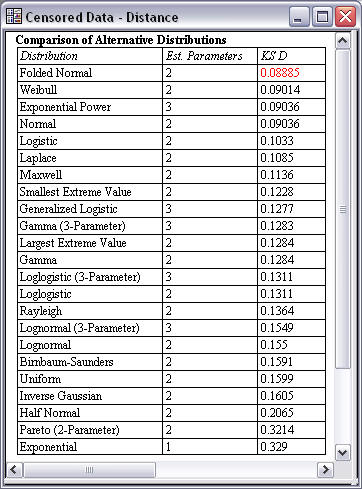
Weibull Analysis
Experience has shown that
failure data can often be well modeled by a Weibull
distribution. A common method to check the fit of a Weibull
distribution is through a Weibull plot. Uncensored failure
times should fall approximately along a straight line.
In the STATGRAPHICS
Weibull plot, you may add a histogram of censored
failure times and confidence limits for failure percentiles.
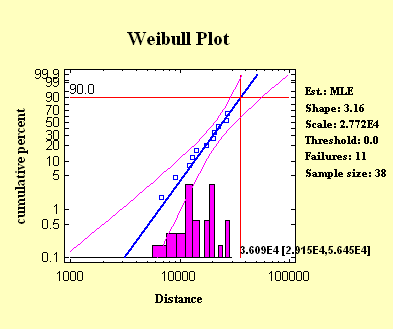
Arrhenius Plot
When failures do not
occur often enough under normal operating conditions, it is
necessary to accelerate the failures by increasing the
stress caused by one or more variables. A very common
accelerant is temperature. By analyzing failure rates at
high temperatures and fitting an Arrhenius model, it is
often possible to extrapolate the data back to a normal
operating temperature (usually expressed in Kelvin).
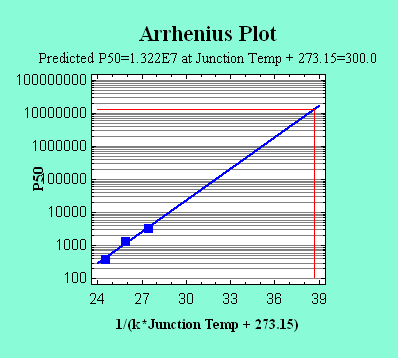
Life Data Regression
To describe the impact of
external variables on failure times, regression models may
be fit. Unfortunately, standard least squares techniques do
not work well for two reasons: the data are often censored,
and the failure time distribution is rarely Gaussian. For
this reason, STATGRAPHICS provides a special procedure that
will fit life data regression models with censoring,
assuming either an exponential, extreme value, logistic,
loglogistic, lognormal, normal or Weibull distribution.
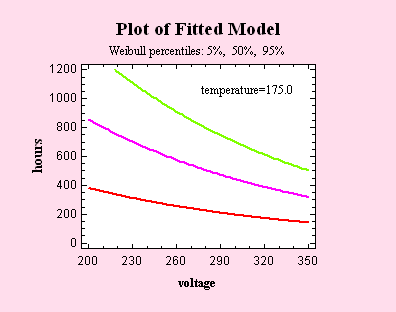
Cox Proportional Hazards
The Cox proportional
hazards procedure is an alternative method for fitting a
life data regression without assuming any specific
distributional form. Instead, it is assumed that the
predictor variables affect the hazard function in a
multiplicative manner. Like the parametric life data
regression procedure, the predictor variables can be either
quantitative or categorical.
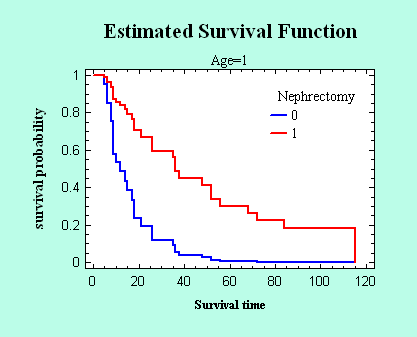
|