Many types of data are
collected over time. Stock prices, sales volumes, interest
rates, and quality measurements are typical examples.
Because of the sequential nature of the data, special
statistical techniques that account for the dynamic nature
of the data are required.
STATGRAPHICS Centurion
provides an extensive set of procedures designed for
analyzing time series data:
1.
Descriptive Methods - time
sequence plots, autocorrelation functions, partial
autocorrelation functions, periodograms, and
cross-correlation functions are all important tools for
characterizing time series data.
2.
Smoothing - a variety of
smoothers are available to estimate the underlying trend
in a time series.
3.
Seasonal Decomposition -
decomposes time series data into trend, cycle, seasonal,
and irregular components, and returns seasonally
adjusted data if desired.
4.
Forecasting - creation of
forecasts beyond the end of the data, using trend
models, moving averages, exponential smoothers, or ARIMA
models.
5.
Automatic Forecasting -
selects the best forecasting method for a time series by
optimizing a specified information criterion.
Descriptive Methods
Characterizing a time
series involves estimating not only a mean and standard
deviation but also the correlations between observations
separated in time. Tools such as the autocorrelation
function are important for displaying the manner in which
the past continues to affect the future. Other tools, such
as the periodogram, are useful when the data contain
oscillations at specific frequencies.
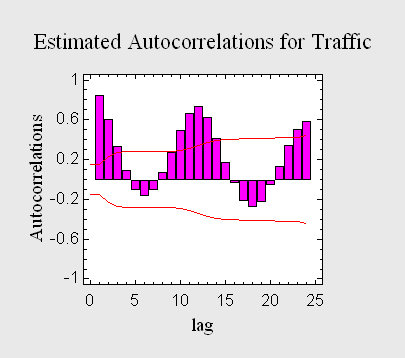
Smoothing
When a time series
contains a large amount of noise, it can be difficult to
visualize any underlying trend. Various linear and nonlinear
smoothers are provided to separate the signal from the
noise.
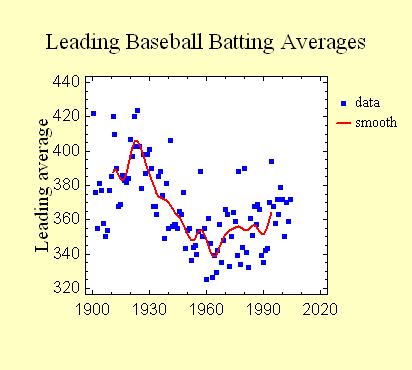
Seasonal Decomposition
When the data contain a
strong seasonal effect, it is often important to separate
the seasonality from the other components in the time
series. This enables one to estimate the seasonal patterns
and to generate seasonally adjusted data.
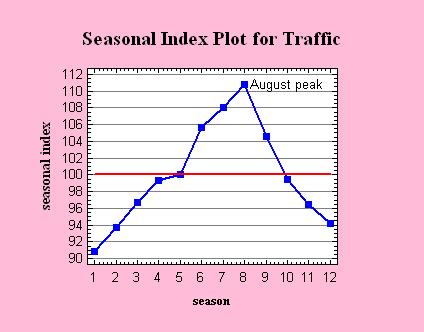
Forecasting
A common goal of time
series analysis is extrapolating past behavior into the
future. The STATGRAPHICS forecasting procedures include
random walks, moving averages, trend models, simple, linear,
quadratic, and seasonal exponential smoothing, and ARIMA
parametric time series models. Users may compare various
models by withholding samples at the end of the time series
for validation purposes.
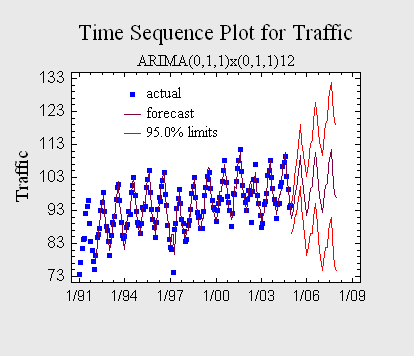
Automatic Forecasting
If desired, users may
elect to let STATGRAPHICS select a forecasting model for
them by comparing multiple models and automatically picking
the model that maximizes a specified information criterion.
The available criteria are based on the mean squared
forecast error, penalized for the number of model parameters
that must be estimated from the data. A common use of this
procedure in Six Sigma is to select an ARIMA model on which
to base an ARIMA control chart, which unlike most control
charts does not assume independence between successive
measurements. In such cases, the analyst may elect to
consider only models of the ARMA(p,p-1) form, which theory
suggests can characterize many dynamic processes.
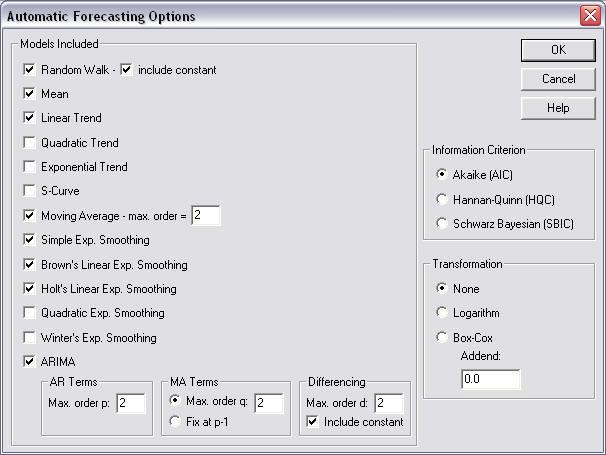 |